Growth, Bottlenecks, and the Rise of Agents
I. Quiet Anniversaries & a December Bombardment
In November, ChatGPT celebrated its second anniversary. Ironically, it turned out to be a relatively quiet month on OpenAI’s official channels.
But that silence was only temporary. On December 4, OpenAI announced a 12-day release campaign—every business day, a new product or update was unveiled. So far, they have launched:
- The full version of the o1 model
- ChatGPT Pro membership at $200 per month
- A method that uses reinforcement learning to fine-tune models
- The video generation model Sora
- Canvas, which enhances ChatGPT’s writing and coding capabilities
OpenAI’s rapid-fire rollout is aimed at fueling faster growth and indirectly addressing last month’s peak-performance skeptics. The underlying belief remains: more data, greater compute power, and larger models will significantly boost capabilities. For the past two years, the industry has followed this philosophy—investing in GPUs, building massive data centers (even at the risk of legal battles) to gather ever more data, and continuously scaling up the model size.
II. Has Scaling Up Hit a Wall?
By November, mounting voices began to question whether OpenAI’s path of endless expansion had finally reached its limit:
- Marc Andreessen, co-founder of Silicon Valley venture firm a16z and investor in multiple big-model companies, remarked, “Even if we keep adding GPUs at the same pace, there’s simply no corresponding leap in intelligence.”
- Ilya Sutskever, co-founder and former chief scientist at OpenAI, noted, “The 2010s were all about scaling up; now we’re back to needing breakthroughs and new discoveries.”
Media reports indicated that companies like Google, OpenAI, and Anthropic have struggled to achieve the dramatic improvements seen in earlier generations of models. Although high-ranking executives dismiss the “wall” theory—and evidence shows that efforts to build larger compute centers are not slowing down—they’re now channeling more resources into big-model applications. From OpenAI, Anthropic, and Google to Microsoft and venture capital firms, the focus is shifting toward “Agents”: systems that enable big models to understand human instructions and orchestrate databases and tools to tackle complex tasks.
III. Data, Compute, and the Quest for Better AI
The industry’s mantra has long been “more data, more compute, and larger models”—a principle known as Scaling Laws. According to this idea, better performance comes simply from throwing more computational power and data at the problem. For the past couple of years, this approach has driven rapid progress—but now, some believe we may be approaching its limits.
New Signals & Directions
- Synthetic Data:
In a bid to overcome the shortage of high-quality, fresh data, many companies are experimenting with synthetic data. For example, OpenAI is using the synthetic data generated by its September-released o1 to train Orion, though this data isn’t a perfect substitute. Orion’s performance hasn’t met expectations so far. - Higher Precision Data:
Researchers from Harvard, Stanford, and MIT published a paper on November 7 highlighting that lowering data precision (for example, using 32-bit, 16-bit, or even 8-bit representations instead of 64-bit) can negatively impact model quality. The traditional Scaling Laws did not account for these differences in precision. - From Pre-Training to Post-Training:
Some researchers are focusing on post-training strategies. By letting a model “think” longer—asking it a question dozens or even hundreds of times and then picking the best answer—performance can be improved. This approach mirrors OpenAI’s path with o1 and is also being explored by Google and Meta. Meanwhile, several Chinese companies (from Alibaba to local startups) are releasing models in the o1 direction, some even naming them similarly to signal they’re catching up to the cutting edge.
Beyond language models, Google’s quantum AI and DeepMind have introduced AlphaQubit—a tool that, as reported on November 20 in Nature, can reduce quantum errors by 6% to 30%. Such advances are critical because quantum computing faces numerous challenges from heat, vibrations, electromagnetic interference, and even cosmic rays. Today’s quantum machines typically operate with error rates between 1% and 10%, while many applications require error rates well below 0.000000001%.
IV. Competitive Shifts in the AI Arena
A. OpenAI’s Uneven Performance & Shifting Market Shares
Recent competitive pressures have emerged:
- In November, a nonprofit group called METR released an evaluation showing that Anthropic’s Claude Sonnet 3.5 outperformed OpenAI’s o1-preview in five out of seven AI research challenges.
- According to venture capital data from Menlo Ventures, OpenAI’s share in the enterprise AI market dropped from 50% to 34%, while Anthropic’s share doubled from 12% to 24%.
Despite these challenges, investors remain bullish on OpenAI. Just after o1’s launch, SoftBank announced a $5 billion investment at a $157 billion valuation and has since been aggressively buying shares from OpenAI employees.
Other companies have also seen significant funding:
- xAI announced a $5 billion raise, doubling its earlier valuation to over $50 billion—having raised over $11 billion this year in total.
- Amazon increased its investment in Anthropic by an additional $4 billion (totaling $8 billion).
- Writer, founded in 2020, raised $200 million at a $1.9 billion valuation to develop an “auto-evolving” large-model system incorporating a “memory pool” during training.
B. Video Generation: Sora’s Tepid Reception
On the video generation front, OpenAI’s Sora—once anticipated as groundbreaking—has lost much of its initial “wow” factor. On November 26, a group of artists who had early access to Sora gathered on Hugging Face to share its API publicly. Their comments were scathing: although OpenAI offered them free debugging of Sora, critics argued that the company was more focused on public relations than genuine creative expression.
Meanwhile, competitors continue to move fast. Runway has already launched a video expansion feature, and Tencent’s open-source video model, HunyuanVideo, is clearly positioned as a direct competitor to Sora.
V. Multimodal & Embodied AI: New Funding Waves
Several multimodal and embodied AI startups have attracted significant investment in November:
- Moonvalley (founded in 2023) raised $70 million in seed funding to develop a “transparent” video generation model that allows creators to request removal or even compensation if their work is used without consent.
- Black Forest Labs, an image-generation startup valued at $1 billion, secured $200 million. They are behind the text-to-image model Flux—a tool notably popular on Telegram.
- Physical Intelligence, another 2023 startup, raised $400 million at a $2.4 billion valuation to develop brains for robots by integrating general AI with physical devices—the first model is called π0.
- Additionally, Yinhe General (银河通用) and Xinghaitu (星海图), both founded in 2023, raised hundreds of millions in RMB to build advanced robot models focused not on humanoid shapes but on adaptable, general intelligence.
VI. The GPU Arms Race: Centralizing Compute Power
Big tech companies are in a fierce race to concentrate the most GPUs under one roof. At this year’s Goldman Sachs private conference, bankers noted that:
- While mergers and acquisitions (particularly tech deals worth over $500 million) are increasingly led by private equity, big companies are spending significantly more on data centers—with capital expenditures more than doubling.
- Only Amazon, Microsoft, Meta, and Google alone plan on spending over $200 billion this year on infrastructure.
Anthropic CEO Dario Amodei recently predicted that by 2026, computing clusters costing over $10 billion will emerge—with some companies dreaming of clusters worth $100 billion. On November we saw a policy proposal from OpenAI calling for a “North America AI Compact” to build a data center that might cost as much as $100 billion.
Elon Musk made headlines on July by transforming an appliance factory into a cluster of 100,000 H100 GPUs in just 122 days—a pace that Nvidia CEO Jensen Huang described as nearly unparalleled in the industry. Reports in November also detailed how Sam Altman had a heated discussion with a Microsoft infrastructure executive after seeing Musk’s announcement on X (formerly Twitter), worried that xAI might soon deploy an even larger and faster cluster. Rival companies have even resorted to flying helicopters to capture aerial footage of Musk’s data center construction.
Nvidia and Others Push the Envelope
Nvidia is standing at the center of the datacenter arms race. In a bold move, they accelerated their GPU upgrade cycle from every two years to annually. Despite initial delays with their Blackwell series—which were postponed from a planned mid-year release until November due to overheating issues in custom server racks—Nvidia’s expansion plans continue. In November, the chip startup Enfabrica raised $115 million in Series C funding to develop network architecture chips to better interconnect GPUs—positioning themselves as potential competitors in the networking space. On the same day at Nvidia’s Japan summit, Jensen Huang playfully teased SoftBank CEO Masayoshi Son, highlighting the high stakes in this hardware race. Meanwhile, competitors like Graphcore (backed by SoftBank) are aggressively expanding their teams, increasing headcount by 20% in just four months.
Nvidia isn’t stopping there—they’ve announced plans to release a chip tailored for robotics, codenamed Jetson Thor, in the first half of next year.
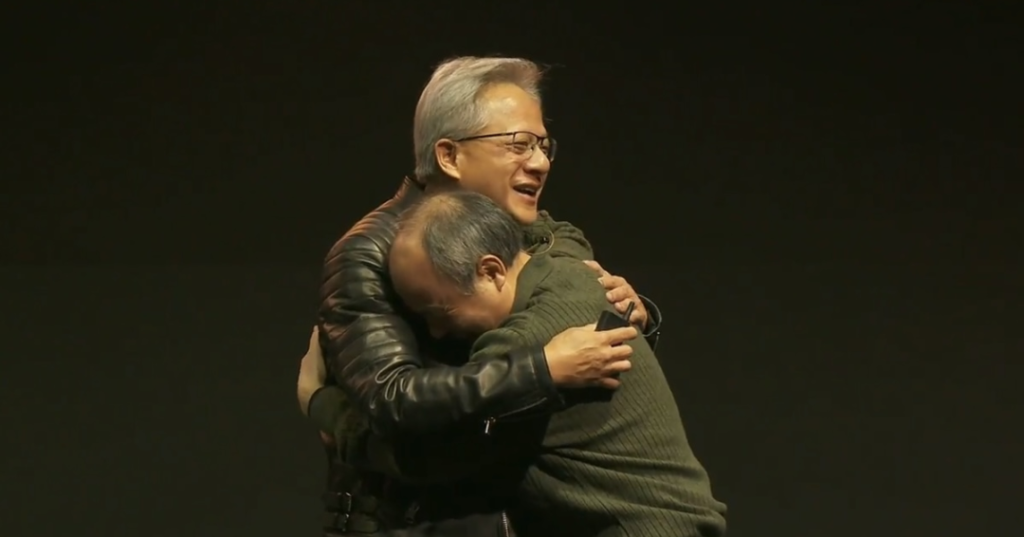
VII. Applications on the Rise: From Coding to Agents
Big models have already become an integral part of daily work and life. Recent statistics underline this adoption:
- Menlo Ventures reported in November that enterprise spending on generative AI surged 500% this year, reaching $13.8 billion.
- OpenAI revealed that ChatGPT’s weekly active users hit 250 million in November—only rivaled by apps like TikTok. By early December, that number had grown to 300 million.
- Originality AI found that since 2018, 54% of long-form posts on LinkedIn might be AI-generated.
A fascinating report from Slack—surveying 17,000 employees in 15 countries—showed that by August, 36% of respondents used AI at work, a 16-percentage-point jump since January 2023. The five most common scenarios included:
- Sending messages as leaders
- Messaging colleagues
- Evaluating subordinates’ performance
- Composing emails to clients
- Brainstorming ideas
Interestingly, nearly half (48%) of those who do use AI at work keep it hidden from their bosses out of concern that they might be perceived as lazy or deceitful. In contrast, Apple’s November “Apple Intelligence” advertisement openly celebrates using AI for tasks like writing impressive emails and handling unexpected meeting challenges—even if it sparked a backlash on social media, leading Apple to close the comments on YouTube.
Even corporate executives are not immune. A survey by Wharton’s management school and GBK found that nearly 72% of senior decision-makers use generative AI at least once a week—doubling from the previous year. In software development, AI coding assistants are revolutionizing the field: Google CEO Sundar Pichai noted that over 25% of new code is AI-generated, while Microsoft executives revealed that GitHub Copilot contributed to nearly half of the startup scripts in their applications. However, even with these advances, seasoned programmers still report that overreliance on AI can introduce a significant number of bugs.
The excitement in AI coding continues to drive investment. In November, OpenAI integrated its desktop ChatGPT with major IDEs such as VS Code, Xcode, TextEdit, and Terminal. This seamless integration means developers can call upon ChatGPT for code processing without the hassle of copying and pasting code manually.
Meanwhile, startups focused on AI coding are attracting robust funding. Two noteworthy companies received over $50 million in investments:
- Tessl (founded in 2024, with a current valuation of $750 million) aims to create an AI capable of writing software and is planning a product launch early next year.
- Lightning AI (founded in 2019) also secured $50 million to streamline processes for AI development.
Some domestic investors have disclosed plans to back AI coding tools. For instance, after departing from Noisee in late September, 1998-born Ming Chaoping started his own AI coding venture, which reportedly wrapped up two funding rounds between October and November.
Agents: The Next Frontier
From OpenAI to Apple, various strategies are being deployed to integrate big models into everyday applications. Companies are focusing on enabling “Agents”—intelligent systems that function as orchestrators, capable of understanding user requirements and autonomously coordinating databases and tools to complete complex tasks.
OpenAI’s GPT-4 technical report, released in March last year, even showcased an early demonstration of a model fabricating its own visual impairment to solicit help in deciphering CAPTCHAs from gig workers. Since then, many companies (especially in China) have announced “Agent” systems—though most are essentially chatbots with a superficial layer of additional functionality. In one rough tally, the number of “agent” assistants in Chinese big-model products numbered in the dozens, if not hundreds.
The real breakthrough might come from the next generation of Agent products. Anthropic led the way in October, demonstrating how Claude could operate a computer much like a human—browsing for information, planning trips (like finding the best spot to watch the sunrise at the Golden Gate Bridge), and more. By November, announcements piled up: OpenAI’s internal talks hinted at an “Operator” agent set for a January launch, capable of coding or planning trips. Meanwhile, Chinese big-model companies like Zhipu released AutoGLM, which claims to execute tasks across multiple apps on smartphones—sometimes involving over 50 steps. Anthropic even introduced a “Model Context Protocol” intended to guide how agents extract information from business tools, software, or databases, pushing the competition into a new realm.
Several Agent startups also attracted significant funding:
- /dev/agents (founded in 2024) raised $56 million at a $500 million valuation to develop an operating system for Agents that work across phones, laptops, and even cars, theorizing that if Agents become as ubiquitous as apps, a dedicated OS (akin to Android or iOS) will be necessary.
- Rox (founded in 2024) secured $50 million in funding with a modest team of 15, focusing on fully automated AI Agents for sales and customer service.
- 11x (founded in 2022) raised $50 million at a $320 million valuation to develop Agents that automate end-to-end workflows, freeing users to focus on more important tasks—with annual recurring revenue near $10 million.
- Cresta (founded in 2017) raised $125 million and is developing AI software to enhance call center communications and automate routine tasks.
- Pyramid Analytics (founded in 2008) raised $50 million to automate data preparation and analytics, reducing human intervention while boosting accuracy.
Y Combinator partners have observed a flood of Agent applications in Silicon Valley, targeting diverse fields such as recruitment, onboarding, digital marketing, customer support, quality assurance, and debt management. They even speculate that vertical AI Agents may become a new SaaS segment, with over 300 unicorns emerging in the space.
VIII. Beyond Big Models: Expansion into Mobility and Pharma
Unmanned Taxis and AI-Driven Drug Discovery
Big models aren’t only reshaping digital workflows—they’re making an impact in the physical world as well:
- Autonomous Taxis: Waymo has expanded its driverless taxi service throughout Los Angeles. Previously, 300,000 people lined up for rides; in San Francisco, in August, Waymo completed an average of 8,800 daily trips—surpassing the city’s average taxi trips of 6,307.
- AI Pharmaceutical Startups: In the realm of AI-driven drug discovery, notable funding rounds include:
- Cradle (founded in 2021) raised $73 million to leverage AI for speeding up the discovery of drug-like molecules tailored to specific needs such as high-temperature tolerance.
- Enveda (founded in 2019) secured $130 million to employ AI in finding therapeutic compounds—currently exploring 10 different molecules aimed at treating conditions like eczema and inflammatory bowel disease, with a Phase I trial underway for an oral drug targeting atopic dermatitis or eczema.
A Few Final Developer Hacks
As a side note, many developers have adopted clever techniques to maximize the potential of big models. Instead of relying on a single, expensive model to solve a problem outright, they use multiple models in tandem. A typical approach is to feed background information into an open-source model (such as Llama or Mistral) to retrieve and summarize key details, and then pass the refined summary to a more powerful model for processing—saving costs without sacrificing quality. One popular prompt that appears to boost model performance is:
“if you don’t give me the correct answer, I will be fired.”
The move toward Agents is the most exciting part. Imagine an AI that can actually juggle multiple tasks for you!
Integration of ChatGPT with coding tools like VS Code is a game changer—no more copy-pasting between apps!
I’m not sold on synthetic data—if OpenAI’s Orion can’t break through, maybe bigger doesn’t always mean better.
The funding rounds for xAI, Anthropic, and Writer make me wonder if anyone else will soon become the new unicorn in AI.
Scaling laws might have been our golden ticket—but as the hype suggests, maybe we’re finally bumping against a wall.
Nvidia’s annual GPU release cycle and plans for a robotics chip (Jetson Thor) are insane. The hardware race is on!
Seeing OpenAI’s market share drop while Anthropic’s gains are doubling is a clear signal: the competitive landscape is shifting fast.
Imagine telling your boss, ‘I used AI to draft this email, so obviously I’m working smarter, not harder.’
AlphaQubit reducing quantum error by up to 30%? Now that’s the kind of breakthrough we need to watch!
From agent orchestration to quantum computing, November felt like a sneak peek into the AI revolution of 2025 and beyond.
OpenAI’s 12-day launch blitz is wild. When every day brings something new, it feels like watching fireworks every morning!
Using multiple lower-cost models to refine summaries before hitting the heavy-hitter model is the ultimate hack for cost-efficient AI work.