Reinventing Cross-Border Autonomous Driving Strategies
Overview
In October this year, the head of autonomous driving in Seattle and Senior Director of Autonomous Driving Products in Seattle at an influential tech company held an annual planning meeting. “We almost reached a consensus instantly,” said George.
At the meeting, George outlined three “guarantees”:
- They will continuously increase investment in intelligent driving;
- They will adhere to an AI-first strategy to empower every product line; and
- They will stay committed to globalization so that their intelligent driving solutions serve users around the world.
George explained that one of his daily ways of communicating with the company’s founder was through a group chat where new software bugs or surprises were reported—and he would address them. Their goals are always perfectly aligned, without need for debate: “We want to provide an L3-like intelligent driver assistance experience to all users at the current L2 BOM cost, regardless of city, driving conditions, or road type.”
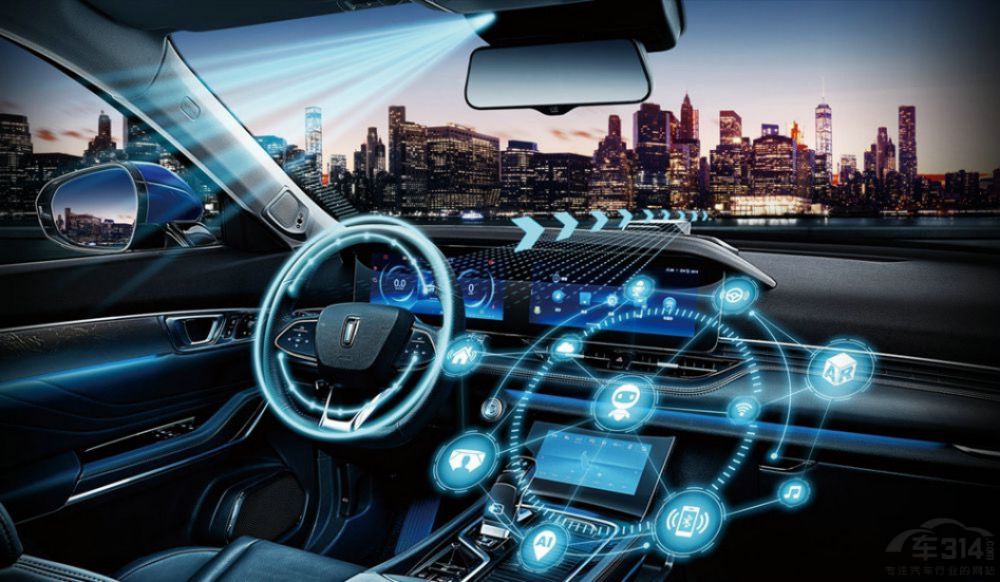
Changing of the Guard and New Challenges
After the former Vice President of Autonomous Driving departed last August, George took over as the top leader of autonomous driving R&D. Within two months he completed an ambitious “Crazy 200 Cities” project, and in January expanded the coverage of their map-free NGP service to 243 cities nationwide.
However, not long afterward, even greater challenges emerged:
- On one hand, Tesla launched its FSD V12 version in North America, pioneering an “end-to-end” approach that reshaped the production algorithm architecture for mass-market smart driving. This shift has once again redirected the technological trends in the industry, and domestic competitors are eyeing a chance to leapfrog.
- On the other hand, the company has experienced significant talent loss in its intelligent driving division. Over the past year, apart from the departing executive, at least five high-level intelligent driving technical managers have joined a rival firm. As one former executive had noted last July, the company’s ability to iterate on its planned smart-driving software releases is not only due to technology but, more importantly, the strength of its teams and internal systems.
Since May this year, the founder has repeatedly emphasized that the company is the first domestic—and one of only two global—automobile makers to mass-produce an end-to-end large AI model (the other being Tesla).
Still, some industry experts question this claim. They note that Tesla’s end-to-end solution uses one unified model for everything from perception to decision-making, whereas domestic players tend to use a “segmented” end-to-end model. Although the decision module replaces some rules with data-driven methods, many rules remain in the process, which some say is not as advanced.
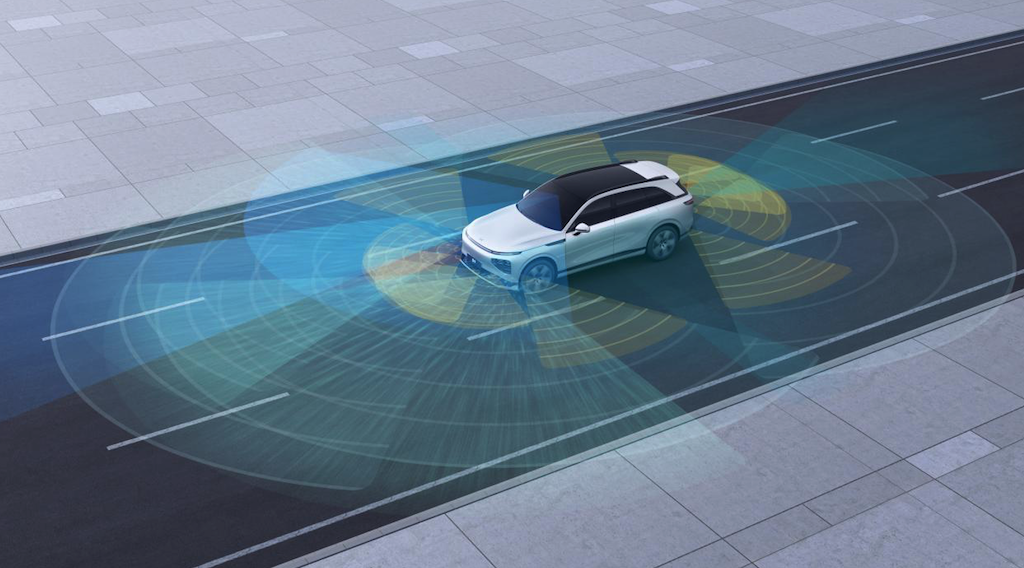
Strategic Adjustments and Talent Reorganization
Quick to sense the crisis, the company swiftly made a series of adjustments in its organizational structure, talent recruitment, and computing resource reserves:
- The R&D team was restructured into three divisions: AI Model Development, AI Application Delivery, and AI Performance.
- They began recruiting senior talent from established U.S. L4 autonomous driving developers such as Cruise and Waymo.
- They ramped up their cloud training resources, planning to expand computing power to over 10 exaFLOPS by the end of next year.
George revealed that the mass-produced end-to-end large model launched in May follows a “one model” architecture because “three networks interlace and overlap, connected by neurons rather than by interfaces defined by rules.”
This week, the company is set to release its Dimensity 5.4.0 system. Compared to the version from five months ago, the new system is expected to upgrade the algorithm architecture and user experience of its smart driving features, potentially shifting the competitive landscape of China’s intelligent driving industry.
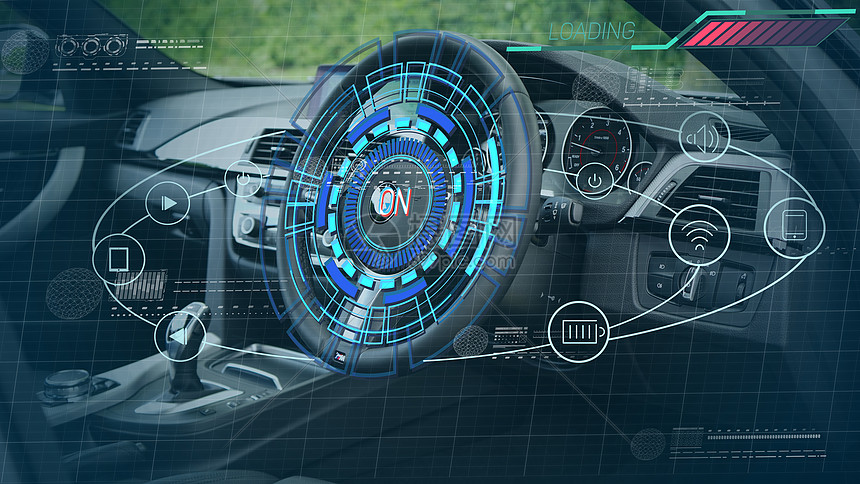
An Exclusive Dialogue
Below is an excerpt from a conversation between Cross border observers: and the company’s executives, discussing how autonomous driving now competes on algorithms, computing power, and data:
Cross border observers:
Autonomous driving is starting to affect Chinese consumers’ car-buying decisions. Based on your observations, how significant is this impact?
George:
Even if ranked outside the top three, intelligent driving is already near the top. For a vehicle priced above 300,000 RMB, lacking high-level autonomous features greatly diminishes its appeal—and this trend is gradually penetrating the 200,000 RMB price segment.
Cross border observers:
For instance, a competitor’s high-end version sees over 50% adoption of its advanced driving package, with one model’s option rate exceeding 70%. What are your current statistics?
George:
Our X9 model’s high-end version currently has an option rate above 73%. Beyond that, we track comprehensive metrics—activation rates of each intelligent driving feature, user penetration, and mileage penetration. In May, after launching our mass-produced end-to-end large model, the monthly user penetration for our urban smart driving service remained at roughly 83%–86%.
Cross border observers:
The X9 starts at over 350,000 RMB. How are adoption rates for lower-priced models?
George:
Starting with our upcoming P7+ launching in early November, high-level intelligent driving will be standard across all models, eliminating the issue of an “option rate.” We’ll deliver a software package that includes all advanced driving features, so every new model will have a 100% adoption rate.
Cross border observers:
Standardizing high-end autonomous driving across all vehicles is a challenging feat. Many competitors can’t pull that off.
George:
Tesla’s success is partly due to its massive fleet and the large amount of training data available. While fleet size is a critical foundation, it doesn’t automatically translate to quality training data—only high-quality driving data is useful. It also demands strong engineering capabilities; sometimes even on-vehicle algorithms or AI are required to ensure the data is effectively utilized.
Additionally, our fleet is diverse—from A-class to C-class vehicles, sedans to SUVs to MPVs—which gives us a rich variety of high-quality data, and enables us to utilize our computing power efficiently. Although we acknowledge there is still a gap with Tesla, we are among the top domestically.
Cross border observers:
Large amounts of data from the fleet require cloud processing. Tesla plans to invest billions in AI chips for its cloud computing, and a competitor has spent over 1 billion RMB in cloud resources, potentially reaching 1 billion USD in the future. What is your investment in cloud computing, and what is the expected growth?
George:
Considering our global competition, by the end of 2025 our cloud computing capacity will exceed 10 exaFLOPS—an increase of 2.6 times compared to our 2024 target. However, we should not just compete on absolute computing power; factors such as quantization and model structure are also critical. We care about both the raw numbers and efficiency—the utilization rate of our cloud training clusters is consistently above 80–90%.
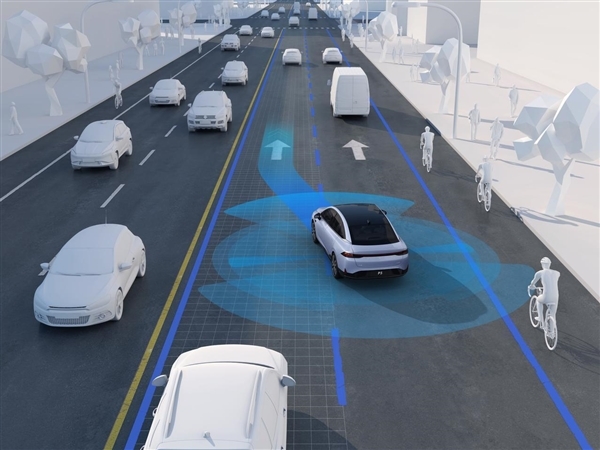
(The dialogue continues through various topics including pricing strategies, sensor choices, data diversity, the evolution of end-to-end architectures, and strategic organizational adjustments.)
For brevity, the interview covers many additional topics—from the challenges of deploying an end-to-end system on vehicle hardware to the importance of a cloud-based foundation model that can be distilled for on-vehicle use, and discussions on future Robotaxi strategies. The executives emphasize that while the shift to an end-to-end approach increases the complexity of the entire system, it is the only path to dramatically advancing from L2 to L3/L4 autonomous driving capabilities.
They also acknowledge the challenges of ensuring that the “end-to-end” approach is truly integrated—moving away from legacy segmented systems towards one unified model, all while maintaining explainability, safety, and efficiency. In essence, the company is betting on a future where its cloud-based foundation models, significantly larger than its current on-vehicle models, will set the ceiling for overall intelligent driving performance, and this will eventually be leveraged across their entire ecosystem of products.
Using a unified architecture where networks interlace instead of using simple rule-based interfaces sounds like next-level engineering.
Their commitment to standardizing high-level autonomous features across all models is a smart strategy to gain market share.
The dialogue about end-to-end being like an iceberg—with only 5% visible—really puts the massive behind-the-scenes work into perspective.
Switching to an end-to-end ‘one model’ approach despite the challenges shows true confidence in their tech.
Their strategy to combine full-managed, semi-managed, and possibly third-party modes all in one platform is innovative.
I’m impressed by how they plan to harness cloud power to scale up computing capacity—10 exaFLOPS is insane!
Doubling product supply through aggressive recruitment? That’s some serious ambition in the cross-border e-commerce space.
The roadmap shift from a segmented model to a full end-to-end approach is daring, but it might just be the game changer.
The emphasis on quality driving data and diversified fleet numbers definitely gives them an edge over competitors.
Reorganizing teams into AI Model Development, Application Delivery, and Performance is a bold move to drive innovation.